Manufacturing is undergoing a digital transformation that involves the implementation of new technologies, processes, and business models. Intelligent manufacturing, also known as digital factory or Industry 4.0, is an emerging field of manufacturing that focuses on using computer-controlled data collection and analysis to automate production processes while also integrating cyber-physical systems such as sensors and networks.
With the advent of AI, ML, and IoT technologies, manufacturers are now able to gather datasets at massive scales; analyze their data with sophisticated algorithms; and use this information to drive their operations in intelligent ways. The convergence of these technologies has enabled many manufacturing companies to implement mechanized production processes that improve operational efficiency and reduce waste.
This article provides an overview of how materials informatics can be used by manufacturers in the age of Intelligent Manufacturing.
What is Materials Informatics?
Materials informatics is an interdisciplinary field that combines computer science, materials science, and engineering to manage, store, and analyze large data sets related to materials and manufacturing processes. Such data may include information about materials properties, specifications, source materials, supplier details, formulation details, batch numbers, sales history, etc.
The data related to materials may also include manufacturing process data such as equipment and tooling information, process parameters, process recipes, and quality data. In other words, materials informatics is the practice of managing and leveraging large amounts of data related to materials and manufacturing processes.
Materials informatics aims to help manufacturers improve their business operations by effectively managing their data assets and leveraging them to create value.
Such value may be added through the execution of data-driven decision-making processes such as automated process parameter tuning, recipe optimization, design-for-manufacturing, and supplier collaboration.
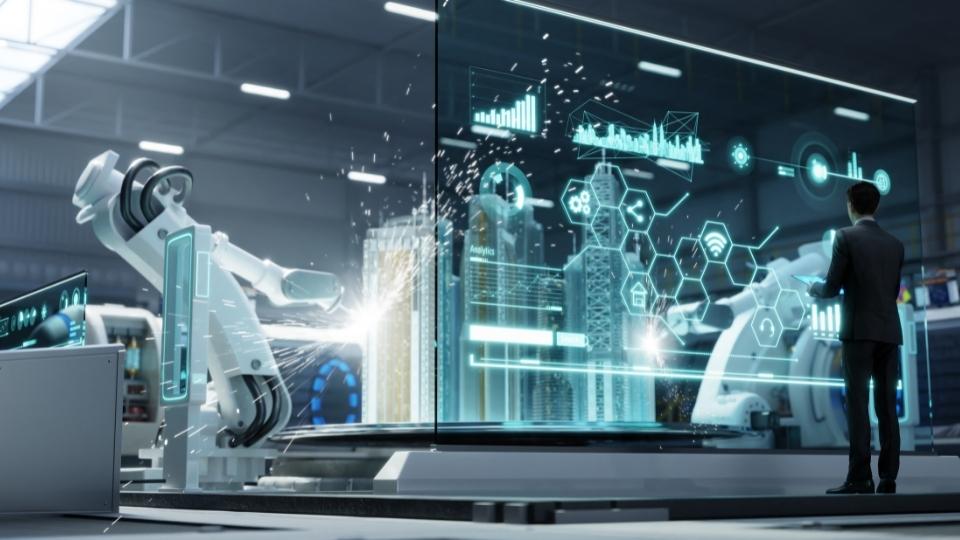
Data and AI in Intelligent Manufacturing
The manufacturing industry is undergoing a digital transformation that is ushering in a new era of production. This transition is expected to provide significant improvements in operational efficiency, product quality, and supply chain management. As part of this digital transformation, manufacturers are now using data and artificial intelligence (AI) to improve their operational performance. The use of AI in manufacturing is not a new concept, but it has received significant attention in recent years due to the advent of new technologies like machine learning, IoT, and blockchain that have made it possible to implement AI in manufacturing environments.
Benefits of implementing ML in manufacturing environments
– Data-driven decision-making: When data is available and can be collected at large scales, it can be leveraged to drive decision-making processes in an automated way. Data-driven decision-making has the potential to improve operational efficiency by eliminating human error and shortening feedback loops in the decision-making process.
– Improved quality: Data can also be used to improve quality by identifying root causes of defects in the supply chain. Data can also be used to improve product design, materials selection, and process design through the use of simulation and optimization tools.
– Reduced costs: When implemented effectively, data-driven decision-making can also help reduce costs by optimizing the supply chain, reducing tooling costs, and eliminating waste in the production process.
– Reduced risk: Data-driven decision-making can also help reduce risk by providing better insights into demand and supply, improving collaboration between stakeholders, and enabling faster response times.

Limitations of Machine Learning in manufacturing environments
– Incomplete data: It is important to note that most manufacturing data is not clean and standardized, which means that it may be difficult to collect, process, and use it for AI purposes. This can pose a challenge for manufacturers who want to implement AI in their operations because they may have to invest heavily in data cleansing and standardization before they can implement AI.
– Inability to understand the data: While data can be used to improve quality and operational efficiency, it can only produce results if it is understood by the machines. Unfortunately, many manufacturers struggle to understand their own data, which means that they may have difficulty in implementing AI in their operations.
– Lack of skilled personnel: Another common challenge in implementing AI in manufacturing environments is the lack of skilled personnel. Many manufacturers may lack the personnel who can effectively collect and use data, implement machine learning algorithms, or build AI-enabled products.
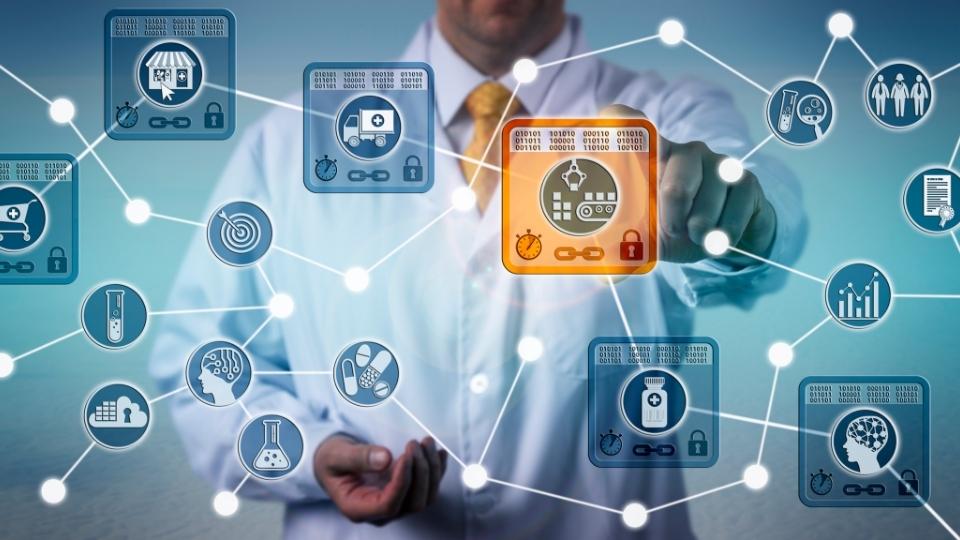
Conclusion
The manufacturing industry has historically been characterized by low levels of automation and manual operations, with humans performing most of the production tasks and using equipment that is outdated and inefficient. However, with advancements in artificial intelligence, machine learning, and Internet of Things technologies, manufacturers can now automate their production processes using smart equipment, data collection, and sophisticated sensors.
Artificial intelligence is helping manufacturers make the switch from manually operated, inefficient production lines to automated production lines that use computer-controlled systems and interconnected networks to drive operations. The use of AI in manufacturing enables manufacturers to collect data from sensors and other equipment, analyze it to identify inefficiencies, and use the insights gained to optimize their operations.
looking for a New PhD Career ?
Join free-ist which is an exclusive network of the world’s top Academia and Corporate Researchers in Science and Technology. We provide access to companies that match you in quality with your skills, as well as compensate more for them.